Methods
In this paper, we developed, to the best of our knowledge, the first multi-image question answering benchmark based on unsupervised anomaly detection. Our framework designs different feature fusion strategies for combing the anomaly map, original image, and PH reconstruction. Besides, inspired by the Querying Transformer (Q-Former), we propose a Knowledge Q-Former (KQ-Former) module to assist the framework in extracting visual features related to textual knowledge. Extensive experiments have been conducted to verify the effectiveness of the framework and proposed KQ-Former module. Additionally, we explore the influence of the anomaly map for the framework in facing unknown anomalies.
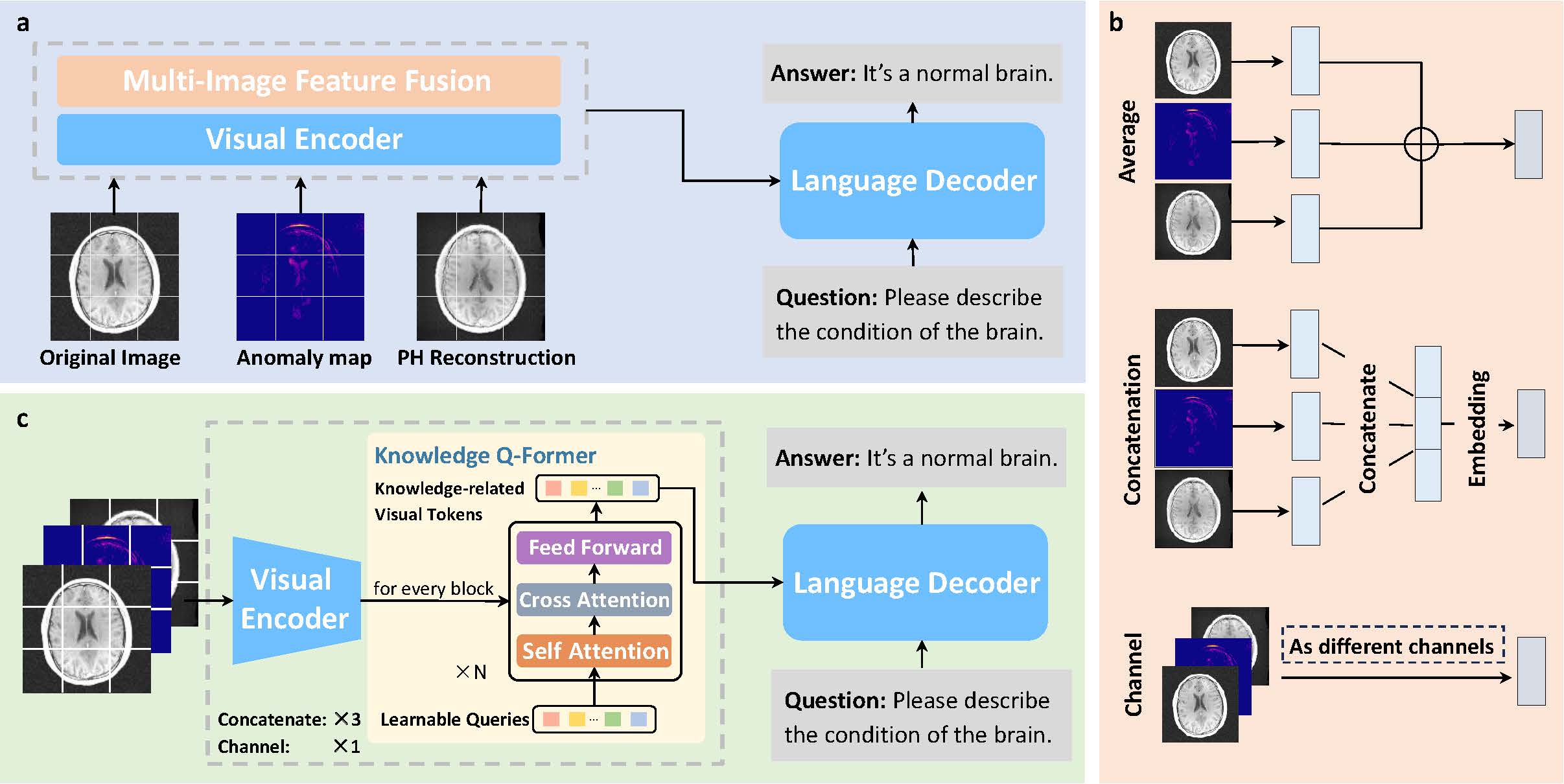